PS:要轉載請註明出處,本人版權所有。
PS: 這個只是基於《我自己》的理解,
如果和你的原則及想法相沖突,請諒解,勿噴。
前置說明
本文作為本人csdn blog的主站的備份。(BlogID=110)
環境說明
- Windows 10
- VSCode
- Python 3.8.10
- Pytorch 1.8.1
- Cuda 10.2
前言
本文是此基礎補全計劃的最終篇,因為從我的角度來說,如果前面這些基礎知識都能夠了解及理解,再加上本文的這篇基礎知識,那麼我們算是小半隻腳踏入了大門。從這個時候,其實我們就已經可以做影象上的基本的分類任務了。除了分類任務,我們還有兩類重要的影象任務是目標檢測和影象分割,這兩項任務都和分類任務有一定的關聯,可以說,分類可以說是這兩類的基礎。
卷積神經網路是一個專門為處理影象資料的網路。下面我們簡單的來看看卷積、池化的含義和怎麼計算的,然後我們通過一個LeNet5的經典網路,訓練一個分類模型。
卷積
卷積是一種運算,類似加減乘除。卷積是一種運算,類似加減乘除。卷積是一種運算,類似加減乘除。重要的事情說三次。
在數學上的定義是:連續n的情況\((f*g)(x) = \int f(n)g(x-n)dn\), 離散n的情況\((f*g)(x) = \sum\limits_{n} f(n)g(x-n)\)。從這裡我們可以看到,卷積就是測量函式f和函式g的翻轉且平移x後的重疊。其二維離散a,b的表達是\((f*g)(x1,x2) = \sum\limits_{a}\sum\limits_{b} f(a, b)g(x1-a, x2-b)\)
卷積是一種運算,類似加減乘除。卷積是一種運算,類似加減乘除。卷積是一種運算,類似加減乘除。重要的事情再說三次。
我們再次想一想,在之前的文章中,我們普遍都建立了一種想法是,把輸入資料拉成一條直線輸入的,這就意味著我們在之前的任務裡面只建立了相鄰輸入資料之間的左右關聯。但是我們可以想一想,是不是所有的資料只建立左右關聯就行了呢?顯而易見的,並不是這樣的,比如我們圖片,可能上下左右4個畫素加起來才是一個貓,如果我們只關聯了左右,那麼它可能是狗或者貓。那麼我們應該通過什麼樣的方式來對圖片畫素的這種二維關聯性進行描述或者計算呢?這種方法就是卷積運算。
卷積網上有許許多多的介紹,大家都做了許多詳細的解答,包含訊號分析、複利、概率以及影象濾波等等方面的解釋。我個人認為我們可以拋開這些方面,從資料之間的關聯性來看這個問題可能是最好理解的,因為我們之前只關注了資料之間左右關聯,我們應該同時關注上下左右的關聯才對,我們要從空間的角度來考慮資料之間的關聯性。而卷積作為一種數學運算,他恰好是計算了資料的上下左右關聯性,因此卷積這種數學運算很適合拿來代替之前的一條線的線性運算。
下面我們來看一下一個基本的卷積計算過程是什麼樣子的。
影象邊緣檢測例項
計算程式碼如下:
def corr2d(X, K): #@save
"""計算⼆維互相關運算。"""
h, w = K.shape
Y = np.zeros((X.shape[0] - h + 1, X.shape[1] - w + 1))
for i in range(Y.shape[0]):
for j in range(Y.shape[1]):
Y[i, j] = (X[i:i + h, j:j + w] * K).sum()
return Y
_X = np.ones((6, 8))
_X[0:2, 2:6] = 0
_X[3:, 2:6] = 0
print(_X)
_K = np.array([[1.0, -1.0]])
_Y = corr2d(_X, _K)
print(_Y)
_Y = corr2d(_X, _K.T)
print(_Y)
結果如圖:
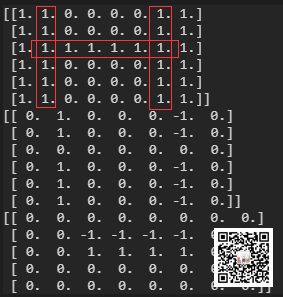
我們可以分別的看到,影象邊緣的數值在經過我們手動構造的濾波器後,成功的檢測到邊緣資訊。
在實際情況中,我們可能要學習邊緣,角點等等特徵,這個時候我們不可能手動去構造我們的濾波器,那麼我們可不可以通過學習的方式把濾波器學習出來呢?下面通過例項來演示:
_X = np.ones((6, 8))
_X[0:2, 2:6] = 0
_X[3:, 2:6] = 0
print(_X)
_K = np.array([[1.0, -1.0]])
_Y = corr2d(_X, _K)
print(_Y)
# _Y = corr2d(_X, _K.T)
# print(_Y)
X = torch.from_numpy(_X)
X.requires_grad = True
X = X.to(dtype=torch.float32)
X = X.reshape(1, 1, 6, 8)
Y = torch.from_numpy(_Y)
Y.requires_grad = True
conv2d = torch.nn.Conv2d(1, 1, (1, 2), bias=False)
for i in range(20):
y_train = conv2d(X)
l = (y_train - Y)**2
conv2d.zero_grad()
# print(l.shape)
l.backward(torch.ones_like(l))
# print(conv2d.weight)
with torch.no_grad():
# print('grad = ', conv2d.weight.grad)
conv2d.weight[:] -= 0.02 * conv2d.weight.grad
# print(conv2d.weight)
# print(conv2d.weight.shape)
if (i + 1) % 2 == 0:
print(f'batch {i+1}, loss {float(l.sum()):.3f}')
print(conv2d.weight)
結果如圖:

我們通過corr2d函式構造出特徵Y,然後我們通過訓練特徵Y,我們可以看到最終卷積層的權重就是接近與1和-1,恰好等於我們構造的特殊濾波器。
這個例項說明了,我們可以通過學習的方式來學習出一個我們想要的濾波器,不需要手動構造。
此外卷積還有卷積核、步長、填充等等資料,我就不造輪子了,網上有很多大佬寫的很好的,大家去看看。此外這裡有個公式非常有用:N=(W-K+2P)/S+1。
池化
我們在上文知道了卷積的輸出結果代表了一片上下左右資料的關聯性,比如一個畫素和之前的9個畫素有關聯,比如一個\(9*9\)的圖,經過一個卷積後,假如還是\(9*9\),這個時候輸出的\(9*9\)裡面的每個畫素我們已經和之前對應位置的一片畫素建立了關聯。但是某些時候,我們希望這種關聯性聚合起來,通過求最大值或者平均等等,這就是池化的概念。以之前例子為例:卷積輸出了\(9*9\)的畫素,經過池化之後,假如變成了\(3*3\),我們可以看到池化輸出的每個畫素代表之前卷積輸出的\(3*3\)個畫素,這代表我們的資訊聚集了,因為一個畫素代表了上一層的多個畫素。
注意池化,我們還可以從視野的角度來看待,還是和上面的例子一樣,假如原圖上的貓是\(9*9\)的畫素,經過卷積池化之後,假如變成了\(3*3\), 這意味著我們從畫素的角度來說,之前81個畫素代表貓,現在9個畫素就可以代表了,也就是之前的一個畫素和現在的一個畫素代表的原圖視野不一樣了,形成了視野放大的感覺。但是有一個缺點就是,這可能導致小目標丟失了,這個在目標檢測裡面會關注到。
一個經典神經網路LeNet5
在2017年12月份,我的這篇文章中《LeNet-5 論文及原理分析(笨鳥角度)》 ( https://blog.csdn.net/u011728480/article/details/78799672 )其實當時我為了學習一些基本知識,也對LeNet5的論文中網路結構部分做了細緻的分析。
注意本文中的C3層和論文中的C3層不一樣。本文的C3層是\(16*6*(5*5+1) = 2496\)個引數。論文原文是\(6*(3*5*5+1)+6*(4*5*5+1)+3*(4*5*5+1)+1* (6*5*5+1)=1516\)個引數。
訓練程式碼如下:
import numpy as np
from numpy.lib.utils import lookfor
import torch
from torchvision.transforms import ToTensor
import os
import torch
from torch import nn
from torch.nn.modules import activation
from torch.nn.modules import linear
from torch.nn.modules.linear import Linear
from torch.utils.data import DataLoader
from torchvision import datasets, transforms
import visdom
vis = visdom.Visdom(env='main')
title = 'LeNet5 on ' + 'FashionMNIST'
legend = ['TrainLoss', 'TestLoss', 'TestAcc']
epoch_plot_window = vis.line(
X=torch.zeros((1, 3)).cpu(),
Y=torch.zeros((1, 3)).cpu(),
win='epoch_win',
opts=dict(
xlabel='Epoch',
ylabel='Loss/Acc',
title=title,
legend=legend
))
def corr2d(X, W): #@save
"""計算⼆維互相關運算。"""
h, w = W.shape
Y = np.zeros((X.shape[0] - h + 1, X.shape[1] - w + 1))
for i in range(Y.shape[0]):
for j in range(Y.shape[1]):
Y[i, j] = (X[i:i + h, j:j + w] * W).sum()
return Y
def TrainConv2d():
_X = np.ones((6, 8))
_X[0:2, 2:6] = 0
_X[3:, 2:6] = 0
print(_X)
_K = np.array([[1.0, -1.0]])
_Y = corr2d(_X, _K)
print(_Y)
# _Y = corr2d(_X, _K.T)
# print(_Y)
X = torch.from_numpy(_X)
X.requires_grad = True
X = X.to(dtype=torch.float32)
X = X.reshape(1, 1, 6, 8)
Y = torch.from_numpy(_Y)
Y.requires_grad = True
conv2d = torch.nn.Conv2d(1, 1, (1, 2), bias=False)
for i in range(20):
y_train = conv2d(X)
l = (y_train - Y)**2
conv2d.zero_grad()
# print(l.shape)
l.backward(torch.ones_like(l))
# print(conv2d.weight)
with torch.no_grad():
# print('grad = ', conv2d.weight.grad)
conv2d.weight[:] -= 0.02 * conv2d.weight.grad
# print(conv2d.weight)
# print(conv2d.weight.shape)
if (i + 1) % 2 == 0:
print(f'batch {i+1}, loss {float(l.sum()):.3f}')
print(conv2d.weight)
class NeuralNetwork(nn.Module):
def __init__(self):
super(NeuralNetwork, self).__init__()
self.lenet5 = nn.Sequential(
# 6*28*28---->6*28*28
nn.Conv2d(1, 6, (5, 5), stride=1, padding=2),
nn.Sigmoid(),
# 6*28*28----->6*14*14
nn.AvgPool2d((2, 2), stride=2, padding=0),
# 6*14*14----->16*10*10
nn.Conv2d(6, 16, (5, 5), stride=1),
nn.Sigmoid(),
# 16*10*10------>16*5*5
nn.AvgPool2d((2, 2), stride=2, padding=0),
nn.Flatten(),
nn.Linear(16*5*5, 1*120),
nn.Sigmoid(),
nn.Linear(1*120, 1*84),
nn.Sigmoid(),
nn.Linear(1*84, 1*10)
)
def forward(self, x):
logits = self.lenet5(x)
return logits
def LoadFashionMNISTByTorchApi():
# 60000*28*28
training_data = datasets.FashionMNIST(
root="..\data",
train=True,
download=True,
transform=ToTensor()
)
# 10000*28*28
test_data = datasets.FashionMNIST(
root="..\data",
train=False,
download=True,
transform=ToTensor()
)
# labels_map = {
# 0: "T-Shirt",
# 1: "Trouser",
# 2: "Pullover",
# 3: "Dress",
# 4: "Coat",
# 5: "Sandal",
# 6: "Shirt",
# 7: "Sneaker",
# 8: "Bag",
# 9: "Ankle Boot",
# }
# figure = plt.figure(figsize=(8, 8))
# cols, rows = 3, 3
# for i in range(1, cols * rows + 1):
# sample_idx = torch.randint(len(training_data), size=(1,)).item()
# img, label = training_data[sample_idx]
# figure.add_subplot(rows, cols, i)
# plt.title(labels_map[label])
# plt.axis("off")
# plt.imshow(img.squeeze(), cmap="gray")
# plt.show()
return training_data, test_data
def train_loop(dataloader, model, loss_fn, optimizer):
size = len(dataloader.dataset)
num_batches = len(dataloader)
loss_sum = 0
for batch, (X, y) in enumerate(dataloader):
# move X, y to gpu
if torch.cuda.is_available():
X = X.to('cuda')
y = y.to('cuda')
# Compute prediction and loss
pred = model(X)
loss = loss_fn(pred, y)
# Backpropagation
optimizer.zero_grad()
loss.backward()
optimizer.step()
loss_sum += loss.item()
if batch % 100 == 0:
loss1, current = loss.item(), batch * len(X)
print(f"loss: {loss1:>7f} [{current:>5d}/{size:>5d}]")
return loss_sum/num_batches
def test_loop(dataloader, model, loss_fn):
size = len(dataloader.dataset)
num_batches = len(dataloader)
test_loss, correct = 0, 0
with torch.no_grad():
for X, y in dataloader:
# move X, y to gpu
if torch.cuda.is_available():
X = X.to('cuda')
y = y.to('cuda')
pred = model(X)
test_loss += loss_fn(pred, y).item()
correct += (pred.argmax(1) == y).type(torch.float).sum().item()
test_loss /= num_batches
correct /= size
print(f"Test Error: \n Accuracy: {(100*correct):>0.1f}%, Avg loss: {test_loss:>8f} \n")
return test_loss, correct
if __name__ == '__main__':
# TrainConv2d()
device = 'cuda' if torch.cuda.is_available() else 'cpu'
print('Using {} device'.format(device))
def init_weights(m):
if type(m) == nn.Linear or type(m) == nn.Conv2d:
nn.init.xavier_uniform_(m.weight)
model = NeuralNetwork()
model.apply(init_weights)
model = model.to(device)
print(model)
batch_size = 200
learning_rate = 0.9
training_data, test_data = LoadFashionMNISTByTorchApi()
train_dataloader = DataLoader(training_data, batch_size, shuffle=True)
test_dataloader = DataLoader(test_data, batch_size, shuffle=True)
loss_fn = nn.CrossEntropyLoss()
optimizer = torch.optim.SGD(model.parameters(), lr=learning_rate)
epochs = 1000
model.train()
for t in range(epochs):
print(f"Epoch {t+1}\n-------------------------------")
train_loss = train_loop(train_dataloader, model, loss_fn, optimizer)
test_loss, test_acc = test_loop(test_dataloader, model, loss_fn)
vis.line(np.array([train_loss, test_loss, test_acc]).reshape(1, 3),
np.ones((1, 3))*t,
win='epoch_win',
update=None if t == 0 else 'append',
opts=dict(
xlabel='Epoch',
ylabel='Loss/Acc',
title=title,
legend=legend
)
)
print("Done!")
# only save param
torch.save(model.state_dict(), 'lenet5.pth')
# save param and net
torch.save(model, 'lenet5-all.pth')
# export onnx
input_image = torch.zeros((1,1,28,28))
input_image = input_image.to(device)
torch.onnx.export(model, input_image, 'model.onnx')
結果如圖:
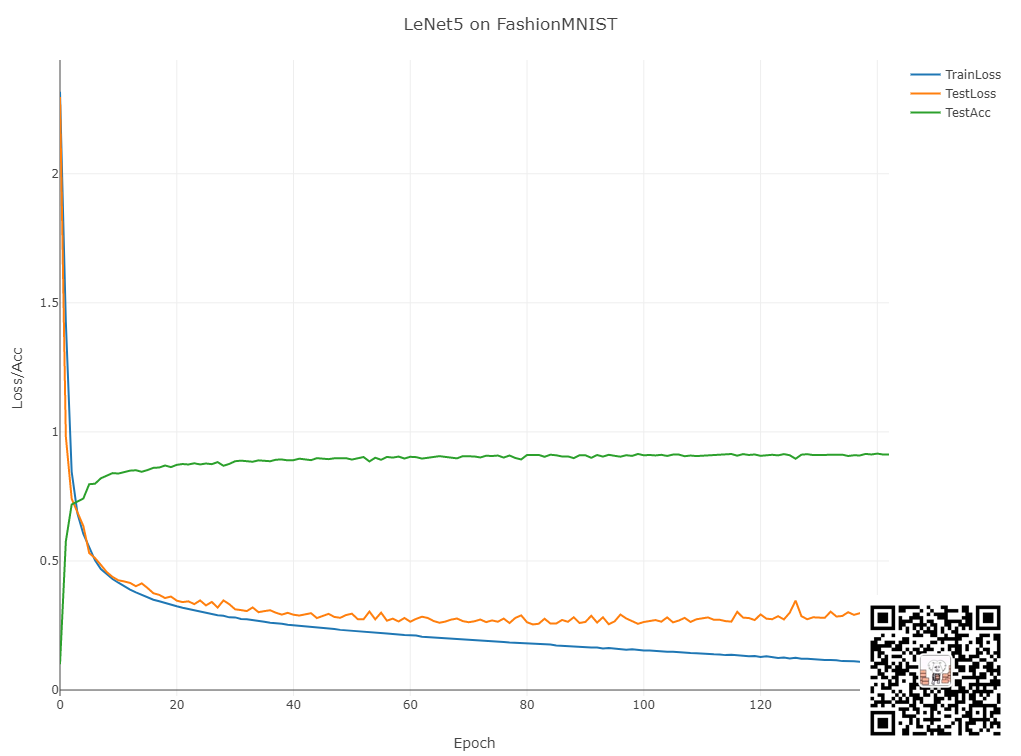
我們從訓練視覺化介面上可以看到,我們的模型確實是收斂了,但是不幸的是準確率大概有90%左右,而且存在過擬合現象。注意這裡我們這個模型,由於有Sigmoid層,導致了很容易出現梯度消失的情況,為了加快訓練,所以學習率設定的很大。
後記
整理本系列的基礎知識的原因是需要加深對深度學習的理解。同時跟著參考資料,重複試驗,重複執行。對我個人而言,只有真實的寫了程式碼之後,才能夠理解的更加透徹。
本文也是此係列的終篇,以後更新隨緣。
參考文獻
- https://github.com/d2l-ai/d2l-zh/releases (V1.0.0)
- https://github.com/d2l-ai/d2l-zh/releases (V2.0.0 alpha1)
- https://blog.csdn.net/u011728480/article/details/78799672 (《LeNet-5 論文及原理分析(笨鳥角度)》)
打賞、訂閱、收藏、丟香蕉、硬幣,請關注公眾號(攻城獅的搬磚之路)
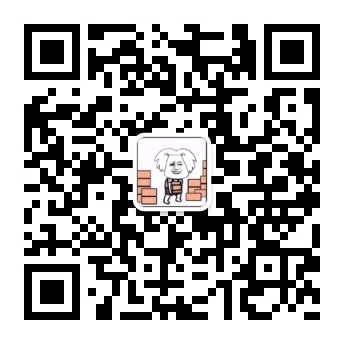
PS: 請尊重原創,不喜勿噴。
PS: 要轉載請註明出處,本人版權所有。
PS: 有問題請留言,看到後我會第一時間回覆。