The curious case of Pagination for Gremlin queries
Why pagination support is hard for the TinkerPop Graph Databases?
Let’s first look at the general requirements for “pagination” support, and then we will analyze the difficulties faced by the graph databases to implement such support.
Pagination Requirement 1. Given a query, a client application may request the results, one page at a time. The most common definitions of a page are: (a) a fixed count of results, (b) a fixed size (in bytes) of the results. Also, note that, there is typically no time limit within which a subsequent page can be requested.
Pagination Requirement 2. The client application may request to skip multiple pages and fetch the following page.
Supporting Pagination Requirement 1: Fetch one page at a time
To return results one page at a time, a database needs to maintain some state, which can later be used to resume the computation for the next page.
In the relational world, such state management is relatively simple. For example, for “Select * from Table” queries, the state can be a pointer to the most recent row that has been returned to the client. With more complex SQL, the state can be a bit more elaborate, but nonetheless very small in size (think bytes). Also, because of the small size of the state, it’s much easier to reload the context and resume the computation for the next page.
However, for graph queries, the state that needs to be managed can be arbitrarily complex and large. This is primarily due to the unstructured and often iterative nature of the graph traversals. To understand this argument, let’s look at an example. Let’s say that the query we need to paginate is: g.V(A).out().out(), with a requested page size of 5.
The figure 1 and figure 2 show the parts of the graph that was visited during the computation of the 1st page and 2nd page respectively, and what state we needed to store for computing their following pages.



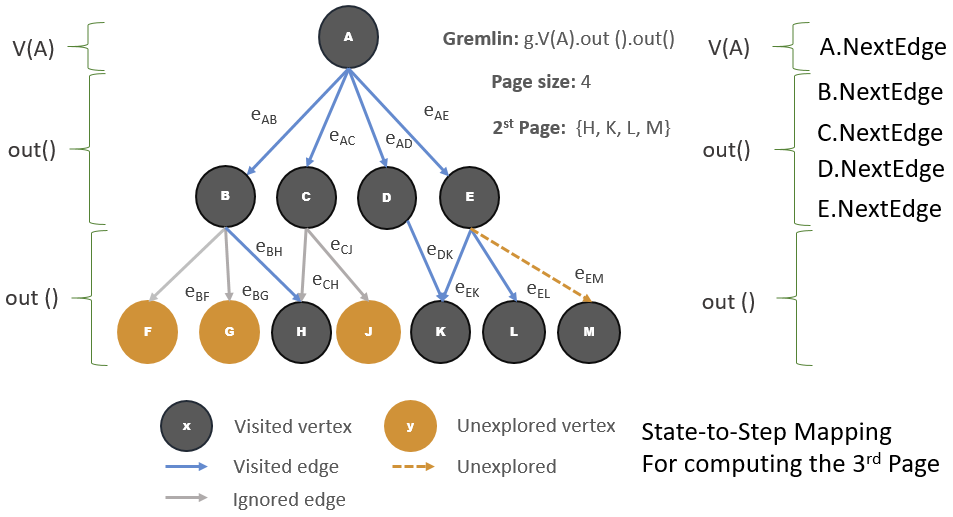
Some of the key observations here are:
Observation 1: The state grows linearly w.r.t. to the number of non-result vertices (or intermediate vertices) visited during a traversal. While this conclusion seems to be very specific to this example, I like to argue that it’s true in general as well. While I didn’t considered deriving a formal proof, the basic intuition lies in the following analogy.
In relational world, “tables are first class citizens” and queries are written by directly referring the tables. We can’t really refer a “row” or “column” directly, and they have to be accessed by applying filtering conditions on tables. This limits the amount of the state one needs to be maintained. For example, when we write a query joining two tables, it is enough to keep one pointer for each table to compute the next page of the join. In other word, the state is roughly proportional to the number of first class citizens referred in the query.
However, in the world of graph databases, “the vertices are first class citizens” (in TinkerPop, edges and properties are as well), and one can directly refer them in the graph queries. Now, analogous to the relational world, when we execute a graph traversal we are effectively conducting a complex join among the vertices that take part in the traversal. In the general case, to support pagination, we need to store some state for each of the participating first class citizen, as each of them may contribute to the final output during the computation of the next page.
When we execute a graph traversal we are effectively conducting a complex join among the vertices that take part in the traversal.
Observation 2: Generating the state for computing the next page is roughly equivalent to running the traversal again. During a graph traversal, when a page worth of results has been generated, we need to compute the state, which can then be be used in the next phase. However, generating the state is non-trivial. We would need to unwind the operator stack to see at state the participating vertices are. Not only that, the state information need to be mapped to the operators in the execution tree. This is because, depending on the complexity of the graph, same node can appear in the same state via multiple paths.
Observation 3: The example shown above is really a very basic graph query on a very structurally well-behaved graph (in this case a tree). Gremlin offers 60 odds different steps including advance constructs like repeat..until, choose (effectively if-then-else), order by, group by, map, fold etc. With all these steps the state management is going to get arbitrarily complex.
Observation 4: In this specific case, some optimization can be done to reduce the pagination state, but that won’t work in the general case. For the given query, we can perform a depth-first traversal and make sure that the edges of a vertices are fetched in a fixed order, to reduce the state. However, this approach will limit parallelism, and eliminate batching opportunities (i.e., executing same step() for multiple vertices at the same time).
While this discussion can go on longer, I think the points discussed so far provide enough material for us to think why supporting pagination in a graph database is a complex beast. More importantly, even if the graph databases supported pagination, it doesn’t seem to be case that it would have saved anything for the client application, both in terms of latency and cost.
Supporting Pagination Requirement 2: Skip pages
Needless to say that this requirement is even more demanding. In this case, keeping around a pointer to the last result is not sufficient. The database needs the ability to efficiently compute the start pointer of a future page.
Again for simple, “Select * from table” queries the computation involves calculating the number of rows that needs to skipped and how to get to the row that will need to be the part of the result.
For graph queries, the starting point of a future page is impossible to compute without actually executing the query and discarding all the results corresponding to the skipped pages. So, supporting this requirement for a graph database won’t save the client application anything.
This summarizes our discussion on the challenges for supporting pagination in graph databases.
PS: In this discussion, we are assuming that computing all the results of a query and storing the results for arbitrarily long period of time, is not a viable solution. Resource governance of supporting such an approach would be very cumbersome. Moreover, the customer would pay a lot of unnecessary cost, especially when only few among the large number of pages are fetched.