Beyond Interactive: Notebook Innovation at Netflix
Beyond Interactive: Notebook Innovation at Netflix
Notebooks have rapidly grown in popularity among data scientists to become the de facto standard for quick prototyping and exploratory analysis. At Netflix, we’re pushing the boundaries even further, reimagining what a notebook can be, who can use it, and what they can do with it. And we’re making big investments to help make this vision a reality.
In this post, we’ll share our motivations and why we find Jupyter notebooks so compelling. We’ll also introduce components of our notebook infrastructure and explore some of the novel ways we’re using notebooks at Netflix.
If you’re short on time, we suggest jumping down to the Use Cases section.
Motivations
Making this possible is no small feat; it requires extensive engineering and infrastructure support. Every day more than 1 trillion events are written into a streaming ingestion pipeline, which is processed and written to a 100PB cloud-native data warehouse. And every day, our users run more than 150,000 jobs against this data, spanning everything from reporting and analysis to machine learning and recommendation algorithms. To support these use cases at such scale, we’ve built an industry-leading Data Platform which is flexible, powerful, and complex (by necessity). We’ve also built a rich ecosystem of complementary tools and services, such as

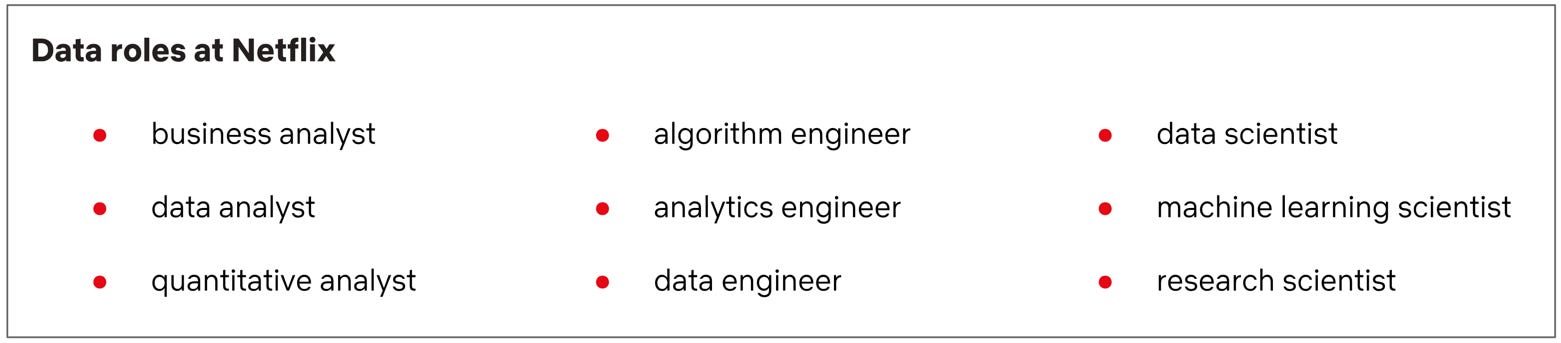
User diversity is exciting, but it comes at a cost: the Netflix Data Platform — and its ecosystem of tools and services — must scale to support additional use cases, languages, access patterns, and more. To better understand this problem, consider 3 common roles: analytics engineer, data engineer, and data scientist.

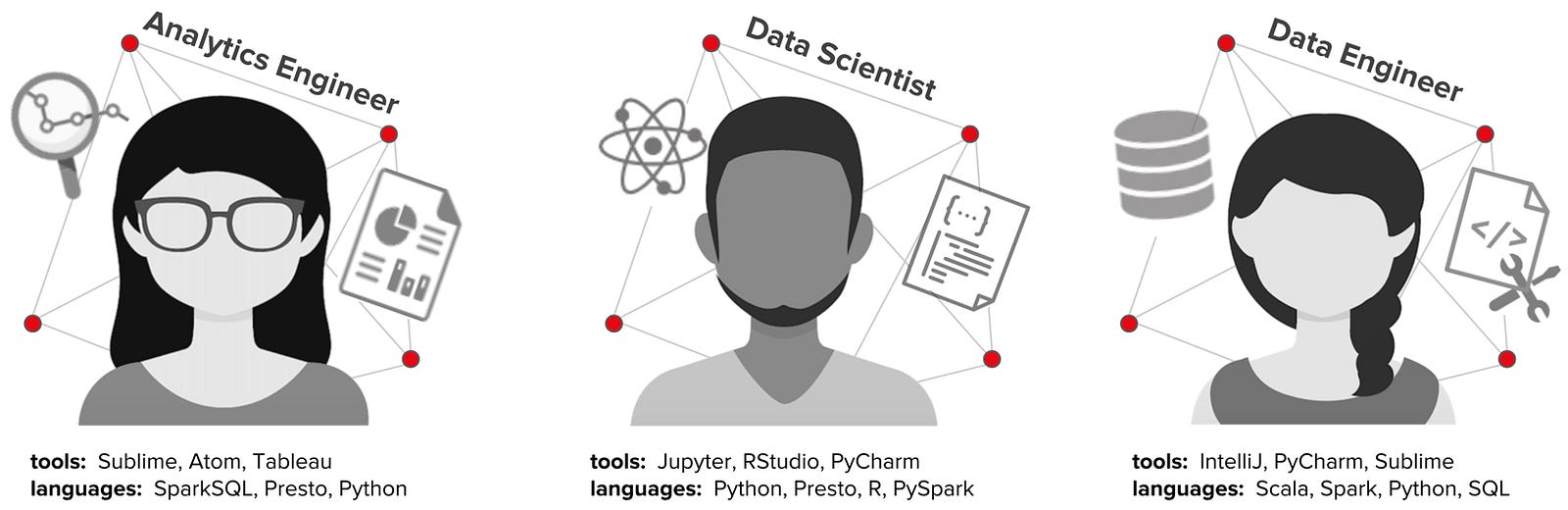
Generally, each role relies on a different set of tools and languages. For example, a data engineer might create a new aggregate of a dataset containing trillions of streaming events — using Scala in IntelliJ. An analytics engineer might use that aggregate in a new report on global streaming quality — using SQL and Tableau. And that report might lead to a data scientist building a new streaming compression model — using R and RStudio. On the surface, these seem like disparate, albeit complementary, workflows. But if we delve deeper, we see that each of these workflows has multiple overlapping tasks:
data exploration — occurs early in a project; may include viewing sample data, running queries for statistical profiling and exploratory analysis, and visualizing data
data preparation — iterative task; may include cleaning, standardizing, transforming, denormalizing, and aggregating data; typically the most time-intensive task of a project
data validation — recurring task; may include viewing sample data, running queries for statistical profiling and aggregate analysis, and visualizing data; typically occurs as part of data exploration, data preparation, development, pre-deployment, and post-deployment phases
productionalization — occurs late in a project; may include deploying code to production, backfilling datasets, training models, validating data, and scheduling workflows
To help our users scale, we want to make these tasks as effortless as possible. To help our platform scale, we want to minimize the number of tools we need to support. But how? No single tool could span all of these tasks; what’s more, a single task often requires multiple tools. When we add another layer of abstraction, however, a common pattern emerges across tools and languages: run code, explore data, present results.
As it happens, an open source project was designed to do precisely that: Project Jupyter.
Jupyter Notebooks
Project Jupyter began in 2014 with a goal of creating a consistent set of open-source tools for scientific research, reproducible workflows, computational narratives, and data analytics. Those tools translated well to industry, and today Jupyter notebooks have become an essential part of the data scientist toolkit. To give you a sense of its impact, Jupyter was awarded the 2017 ACM Software Systems Award — a prestigious honor it shares with Java, Unix, and the Web.
To understand why the Jupyter notebook is so compelling for us, consider the core functionality it provides:
- a messaging protocol for introspecting and executing code which is language agnostic
- an editable file format for describing and capturing code, code output, and markdown notes
- a web-based UI for interactively writing and running code as well as visualizing outputs
The Jupyter protocol provides a standard messaging API to communicate with kernels that act as computational engines. The protocol enables a composable architecture that separates where content is written (the UI) and where code is executed (the kernel). By isolating the runtime from the interface, notebooks can span multiple languages while maintaining flexibility in how the execution environment is configured. If a kernel exists for a language that knows how to communicate using the Jupyter protocol, notebooks can run code by sending messages back and forth with that kernel.
Backing all this is a file format that stores both code and results together. This means results can be accessed later without needing to rerun the code. In addition, the notebook stores rich prose to give context to what’s happening within the notebook. This makes it an ideal format for communicating business context, documenting assumptions, annotating code, describing conclusions, and more.
Use Cases
Of our many use cases, the most common ways we’re using notebooks today are: data access, notebook templates, and scheduling notebooks.
Data Access
Notebooks were first introduced at Netflix to support data science workflows. As their adoption grew among data scientists, we saw an opportunity to scale our tooling efforts. We realized we could leverage the versatility and architecture of Jupyter notebooks and extend it for general data access. In Q3 2017 we began this work in earnest, elevating notebooks from a niche tool to a first-class citizen of the Netflix Data Platform.
From our users’ perspective, notebooks offer a convenient interface for iteratively running code, exploring output, and visualizing data — all from a single cloud-based development environment. We also maintain a Python library that consolidates access to platform APIs. This means users have programmatic access to virtually the entire platform from within a notebook. Because of this combination of versatility, power, and ease of use, we’ve seen rapid organic adoption for all user types across our entire platform.
Today, notebooks are the most popular tool for working with data at Netflix.
Notebook Templates
As we expanded platform support for notebooks, we began to introduce new capabilities to meet new use cases. From this work emerged parameterized notebooks. A parameterized notebook is exactly what it sounds like: a notebook which allows you to specify parameters in your code and accept input values at runtime. This provides an excellent mechanism for users to define notebooks as reusable templates.
Our users have found a surprising number of uses for these templates. Some of the most common ones are:
- Data Scientist: run an experiment with different coefficients and summarize the results
- Data Engineer: execute a collection of data quality audits as part of the deployment process
- Data Analyst: share prepared queries and visualizations to enable a stakeholder to explore more deeply than Tableau allows
- Software Engineer: email the results of a troubleshooting script each time there’s a failure
Scheduling Notebooks
One of the more novel ways we’re leveraging notebooks is as a unifying layer for scheduling workflows.
Since each notebook can run against an arbitrary kernel, we can support any execution environment a user has defined. And because notebooks describe a linear flow of execution, broken up by cells, we can map failure to particular cells. This allows users to describe a short narrative of execution and visualizations that we can accurately report against when running at a later point in time.
This paradigm means we can use notebooks for interactive work and smoothly move to scheduling that work to run recurrently. For users, this is very convenient. Many users construct an entire workflow in a notebook, only to have to copy/paste it into separate files for scheduling when they’re ready to deploy it. By treating notebooks as a logical workflow, we can easily schedule it the same as any other workflow.
We can schedule other types of work through notebooks, too. When a Spark or Presto job executes from the scheduler, the source code is injected into a newly-created notebook and executed. That notebook then becomes an immutable historical record, containing all related artifacts — including source code, parameters, runtime config, execution logs, error messages, and so on. When troubleshooting failures, this offers a quick entry point for investigation, as all relevant information is colocated and the notebook can be launched for interactive debugging.
Notebook Infrastructure
Supporting these use cases at Netflix scale requires extensive supporting infrastructure. Let’s briefly introduce some of the projects we’ll be talking about.
nteract is a next-gen React-based UI for Jupyter notebooks. It provides a simple, intuitive interface and offers several improvements over the classic Jupyter UI, such as inline cell toolbars, drag and droppable cells, and a built-in data explorer.
Papermill is a library for parameterizing, executing, and analyzing Jupyter notebooks. With it, you can spawn multiple notebooks with different parameter sets and execute them concurrently. Papermill can also help collect and summarize metrics from a collection of notebooks.
Commuter is a lightweight, vertically-scalable service for viewing and sharing notebooks. It provides a Jupyter-compatible version of the contents API and makes it trivial to read notebooks stored locally or on Amazon S3. It also offers a directory explorer for finding and sharing notebooks.
Titus is a container management platform that provides scalable and reliable container execution and cloud-native integration with Amazon AWS. Titus was built internally at Netflix and is used in production to power Netflix streaming, recommendation, and content systems.
We explore this architecture in our follow-up blog post, Scheduling Notebooks at Netflix. For the purposes of this post, we’ll just introduce three of its fundamental components: storage, compute, and interface.