機器學習-實戰-入門-linearSVC和SVC,身高體重與胖瘦關係的分類與預測
阿新 • • 發佈:2019-01-24
作者:橘子派
宣告:版權所有,轉載請註明出處,謝謝。
實驗環境:
Windows10
Sublime
Anaconda 1.6.0
Python3.6
根據現有身高和體重訓練模型,再對測試集做出判斷
程式碼功能
一.根據現有資料建立標籤
二.資料資訊視覺化繪圖
執行結果
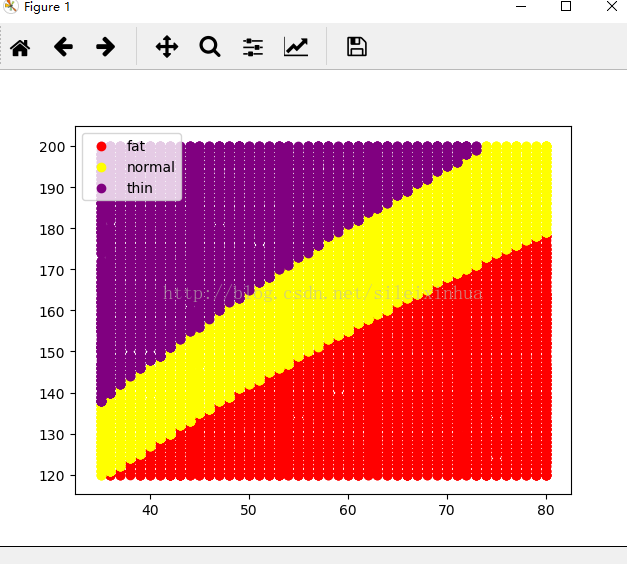
三.運用sklearn中的SVM的SVC訓練資料並預測結果
執行結果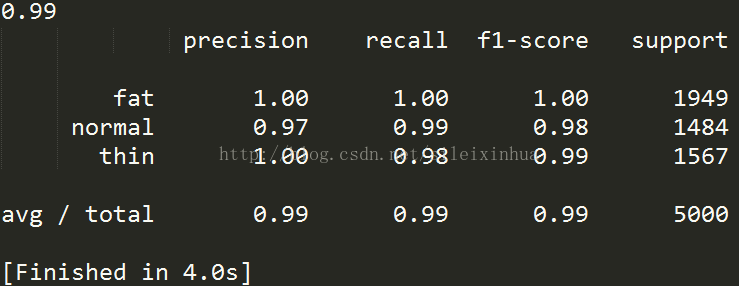
交叉驗證三組測試集平均預測精度為0.99
四.運用sklearn中的SVM的linearSVC訓練資料並預測結果
執行結果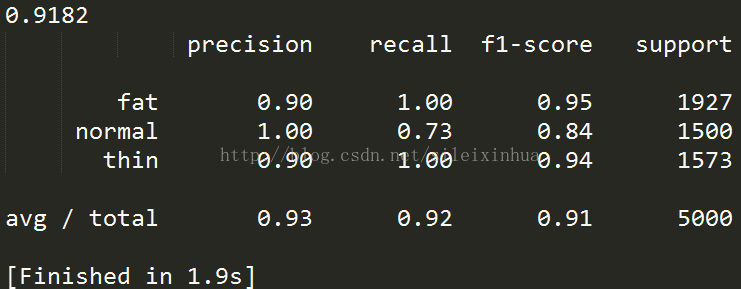
交叉驗證三組測試集平均預測精度為0.9182
參考文獻:
《統計學習方法》
《Web scraping and machine learning by python》
#對資料集進行預處理 import random def calc_bmi(h, w): bmi = w / (h/100) ** 2 if bmi < 18.5: return "thin" if bmi < 25.0: return "normal" return "fat" #bim值小於18.5是瘦,小於25.0是普通,否側是胖 fp = open("./data/bmi.csv","w",encoding="utf-8") fp.write("height,weight,label\r\n") cnt = {"thin":0, "normal":0, "fat":0} for i in range(20000): h = random.randint(120,200) w = random.randint(35, 80) label = calc_bmi(h, w) cnt[label] += 1 fp.write("{0},{1},{2}\r\n".format(h, w, label)) fp.close() print("ok,", cnt)
二.資料資訊視覺化繪圖
#繪製三種不同型別的資料分佈 import matplotlib.pyplot as plt import pandas as pd tbl = pd.read_csv("./data/bmi.csv", index_col=2) #讀取資料 fig = plt.figure() ax = fig.add_subplot(1, 1, 1) #三種子圖重疊 def scatter(lbl, color): b = tbl.loc[lbl] ax.scatter(b["weight"],b["height"], c=color, label=lbl) scatter("fat", "red") scatter("normal", "yellow") scatter("thin", "purple") #設定不同屬性顏色 ax.legend() plt.show() #顯示影象
執行結果
三.運用sklearn中的SVM的SVC訓練資料並預測結果
#用sklearn的SVC方法來訓練資料集,並交叉驗證預測精度 from sklearn import cross_validation, svm, metrics import matplotlib.pyplot as plt import pandas as pd tbl = pd.read_csv("./data/bmi.csv") #讀取資料 label = tbl["label"] #讀取資料中的標籤列 w = tbl["weight"] / 100 h = tbl["height"] / 200 wh = pd.concat([w, h], axis=1) data_train, data_test, label_train, label_test = cross_validation.train_test_split(wh, label) #將資料分成兩組資料集和測試集 clf = svm.SVC() clf.fit(data_train, label_train) #訓練資料 predict = clf.predict(data_test) #預測資料 ac_score = metrics.accuracy_score(label_test, predict) #生成測試精度 cl_report = metrics.classification_report(label_test, predict) #生成交叉驗證的報告 print(ac_score) #顯示資料精度 print(cl_report) #顯示交叉驗證資料集報告
執行結果
#用sklearn的LinearSVC方法來訓練資料集,並交叉驗證預測精度
from sklearn import cross_validation, svm, metrics
import matplotlib.pyplot as plt
import pandas as pd
tbl = pd.read_csv("./data/bmi.csv")
#讀取資料
label = tbl["label"]
#讀取資料中的標籤列
w = tbl["weight"] / 100
h = tbl["height"] / 200
wh = pd.concat([w, h], axis=1)
data_train, data_test, label_train, label_test = cross_validation.train_test_split(wh,label)
#將資料分成兩組資料集和測試集
clf = svm.LinearSVC()
clf.fit(data_train, label_train)
#訓練資料
predict = clf.predict(data_test)
#預測資料
ac_score = metrics.accuracy_score(label_test, predict)
#生成測試精度
cl_report = metrics.classification_report(label_test, predict)
#生成交叉驗證的報告
print(ac_score)
#顯示資料精度
print(cl_report)
#顯示交叉驗證資料集報告
執行結果