Tensorflow2 自定義資料集圖片完成圖片分類任務
阿新 • • 發佈:2020-06-22
對於自定義資料集的圖片任務,通用流程一般分為以下幾個步驟:
- Load data
- Train-Val-Test
- Build model
- Transfer Learning
其中大部分精力會花在資料的準備和預處理上,本文用一種較為通用的資料處理手段,並通過手動構建,簡單模型, 層數較深的resnet網路,和基於VGG19的遷移學習。
你可以通過這個例子,快速搭建網路,並訓練處一個較為滿意的結果。
# 1. Load data
資料集來自Pokemon的5分類資料, 每一種的圖片數量為200多張,是一個較小型的資料集。
官方專案連結:
https://www.pyimagesearch.com/2018/04/16/keras-and-convolutional-neural-networks-cnns/
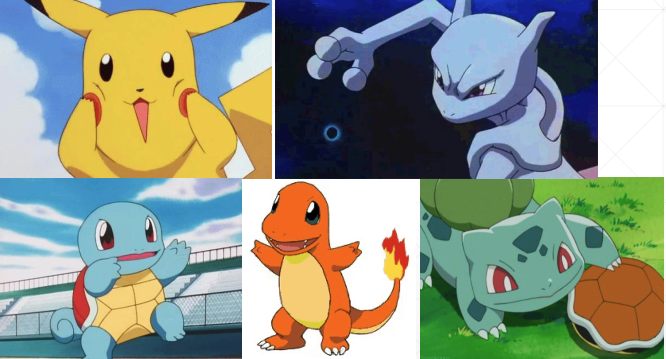
## 1.1 資料集介紹
Pokemon資料夾中包含5個子檔案,其中每個子資料夾名為對應的類別名。資料夾中包含有png, jpeg的圖片檔案。
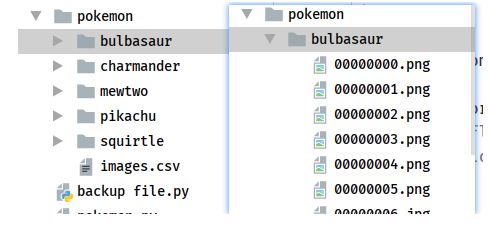
## 1.2 解題思路
- 由於資料夾中沒有劃分,訓練集和測試集,所以需要構建一個csv檔案讀取所有的檔案,及其類別
- shuffle資料集以後,劃分Train_val_test
- 對資料進行預處理, 資料標準化,資料增強, 視覺化處理
"""python
# 建立數字編碼表
import os
import glob
import random
import csv
import tensorflow as tf
from tensorflow import keras
import matplotlib.pyplot as plt
import time
def load_csv(root, filename, name2label):
"""
將分散在各資料夾中的圖片, 轉換為圖片和label對應的一個dataset檔案, 格式為csv
:param root: 檔案路徑(每個子資料夾中的檔案屬於一類)
:param filename: 檔名
:param name2label: 類名編碼表 {'類名1':0, '類名2':1..}
:return: images, labels
"""
# 判斷是否csv檔案已經生成
if not os.path.exists(os.path.join(root, filename)): # join-將路徑與檔名何為一個路徑並返回(沒有會生成新路徑)
images = [] # 存的是檔案路徑
for name in name2label.keys():
# pokemon\pikachu\00000001.png
# glob.glob() 利用萬用字元檢索路徑內的檔案,類似於正則表示式
images += glob.glob(os.path.join(root, name, '*')) # png, jpg, jpeg
print(name2label)
print(len(images), images)
random.shuffle(images)
with open(os.path.join(root, filename), 'w', newline='') as f:
writer = csv.writer(f)
for img in images:
name = img.split(os.sep)[1] # os.sep 表示分隔符 window-'\\' , linux-'/'
label = name2label[name] # 0, 1, 2..
# 'pokemon\\bulbasaur\\00000000.png', 0
writer.writerow([img, label]) # 如果不設定newline='', 2個數據會分為2行寫
print('write into csv file:', filename)
# 讀取現有檔案
images, labels = [], []
with open(os.path.join(root, filename)) as f:
reader = csv.reader(f)
for row in reader:
# 'pokemon\\bulbasaur\\00000000.png', 0
img, label = row
label = int(label) # str-> int
images.append(img)
labels.append(label)
assert len(images) == len(labels)
return images, labels
def load_pokemon(root, mode='train'):
"""
# 建立數字編碼表
:param root: root path
:param mode: train, valid, test
:return: images, labels, name2label
"""
name2label = {} # {'bulbasaur': 0, 'charmander': 1, 'mewtwo': 2, 'pikachu': 3, 'squirtle': 4}
for name in sorted(os.listdir(os.path.join(root))):
# sorted() 是為了復現結果的一致性
# os.listdir - 返回路徑下的所有檔案(資料夾,檔案)列表
if not os.path.isdir(os.path.join(root, name)): # 是否為資料夾且是否存在
continue
# 每個類別編碼一個數字
name2label[name] = len(name2label)
# 讀取label
images, labels = load_csv(root, 'images.csv', name2label)
# 劃分資料集 [6:2:2]
if mode == 'train':
images = images[:int(0.6 * len(images))]
labels = labels[:int(0.6 * len(labels))] # len(images) == len(labels)
elif mode == 'valid':
images = images[int(0.6 * len(images)):int(0.8 * len(images))]
labels = labels[int(0.6 * len(labels)):int(0.8 * len(labels))]
else:
images = images[int(0.8 * len(images)):]
labels = labels[int(0.8 * len(labels)):]
return images, labels, name2label
# imagenet 資料集均值, 方差
img_mean = tf.constant([0.485, 0.456, 0.406]) # 3 channel
img_std = tf.constant([0.229, 0.224, 0.225])
def normalization(x, mean=img_mean, std=img_std):
# [224, 224, 3]
x = (x - mean) / std
return x
def denormalization(x, mean=img_mean, std=img_std):
x = x * std + mean
return x
def preprocess(x, y):
# x: path, y: label
x = tf.io.read_file(x) # 2進位制
# x = tf.image.decode_image(x)
x = tf.image.decode_jpeg(x, channels=3) # RGBA
x = tf.image.resize(x, [244, 244])
# data augmentation
# x = tf.image.random_flip_up_down(x)
x = tf.image.random_flip_left_right(x)
x = tf.image.random_crop(x, [224, 224, 3]) # 模型縮減比例不宜過大,否則會增大訓練難度
x = tf.cast(x, dtype=tf.float32) / 255. # unit8 -> float32
# U[0,1] -> N(0,1) # 提高訓練準確度
x = normalization(x)
y = tf.convert_to_tensor(y)
return x, y
def main():
images, labels, name2label = load_pokemon('pokemon', 'train')
print('images:', len(images), images)
print('labels:', len(labels), labels)
# print(name2label)
# .map()函式要位於.batch()之前, 否則 x=tf.io.read_file()會一次讀取一個batch的圖片,從而報錯
db = tf.data.Dataset.from_tensor_slices((images, labels)).map(preprocess).shuffle(1000).batch(32)
# tf.summary()
# 提供了各類方法(支援各種多種格式)用於儲存訓練過程中產生的資料(比如loss_value、accuracy、整個variable),
# 這些資料以日誌檔案的形式儲存到指定的資料夾中。
# 資料視覺化:而tensorboard可以將tf.summary()
# 記錄下來的日誌視覺化,根據記錄的資料格式,生成折線圖、統計直方圖、圖片列表等多種圖。
# tf.summary()
# 通過遞增的方式更新日誌,這讓我們可以邊訓練邊使用tensorboard讀取日誌進行視覺化,從而實時監控訓練過程。
writer = tf.summary.create_file_writer('logs')
for step, (x, y) in enumerate(db):
with writer.as_default():
x = denormalization(x)
tf.summary.image('img', x, step=step, max_outputs=9) # STEP:預設選項,指的是橫軸顯示的是訓練迭代次數
time.sleep(5)
if __name__ == '__main__':
main()
"""
# 2. 構建模型進行訓練
## 2.1 自定義小型網路
由於資料集數量較少,大型網路的訓練中往往會出現過擬合情況,這裡就定義了一個2層卷積的小型網路。
引入early_stopping回撥函式後,3個epoch沒有較大變化的情況下,模型訓練的準確率為0.8547
"""
# 1. 自定義小型網路
model = keras.Sequential([
layers.Conv2D(16, 5, 3),
layers.MaxPool2D(3, 3),
layers.ReLU(),
layers.Conv2D(64, 5, 3),
layers.MaxPool2D(2, 2),
layers.ReLU(),
layers.Flatten(),
layers.Dense(64),
layers.ReLU(),
layers.Dense(5)
])
model.build(input_shape=(None, 224, 224, 3))
model.summary()
early_stopping = EarlyStopping(
monitor='val_loss',
patience=3,
min_delta=0.001
)
model.compile(optimizer=optimizers.Adam(lr=1e-3),
loss=losses.CategoricalCrossentropy(from_logits=True),
metrics=['accuracy'])
model.fit(db_train, validation_data=db_val, validation_freq=1, epochs=100,
callbacks=[early_stopping])
model.evaluate(db_test)
"""
## 2.2 自定義的Resnet網路
resnet 網路對於層次較深的網路的可訓練型提升很大,主要是通過一個identity layer保證了深層次網路的訓練效果不會弱於淺層網路。
其他文章中有詳細介紹resnet的搭建,這裡就不做贅述, 這裡構建了一個resnet18網路, 準確率0.7607。
"""
import os
import numpy as np
import tensorflow as tf
from tensorflow import keras
from tensorflow.keras import layers
tf.random.set_seed(22)
np.random.seed(22)
os.environ['TF_CPP_MIN_LOG_LEVEL'] = '2'
assert tf.__version__.startswith('2.')
class ResnetBlock(keras.Model):
def __init__(self, channels, strides=1):
super(ResnetBlock, self).__init__()
self.channels = channels
self.strides = strides
self.conv1 = layers.Conv2D(channels, 3, strides=strides,
padding=[[0, 0], [1, 1], [1, 1], [0, 0]])
self.bn1 = keras.layers.BatchNormalization()
self.conv2 = layers.Conv2D(channels, 3, strides=1,
padding=[[0, 0], [1, 1], [1, 1], [0, 0]])
self.bn2 = keras.layers.BatchNormalization()
if strides != 1:
self.down_conv = layers.Conv2D(channels, 1, strides=strides, padding='valid')
self.down_bn = tf.keras.layers.BatchNormalization()
def call(self, inputs, training=None):
residual = inputs
x = self.conv1(inputs)
x = tf.nn.relu(x)
x = self.bn1(x, training=training)
x = self.conv2(x)
x = tf.nn.relu(x)
x = self.bn2(x, training=training)
# 殘差連線
if self.strides != 1:
residual = self.down_conv(inputs)
residual = tf.nn.relu(residual)
residual = self.down_bn(residual, training=training)
x = x + residual
x = tf.nn.relu(x)
return x
class ResNet(keras.Model):
def __init__(self, num_classes, initial_filters=16, **kwargs):
super(ResNet, self).__init__(**kwargs)
self.stem = layers.Conv2D(initial_filters, 3, strides=3, padding='valid')
self.blocks = keras.models.Sequential([
ResnetBlock(initial_filters * 2, strides=3),
ResnetBlock(initial_filters * 2, strides=1),
# layers.Dropout(rate=0.5),
ResnetBlock(initial_filters * 4, strides=3),
ResnetBlock(initial_filters * 4, strides=1),
ResnetBlock(initial_filters * 8, strides=2),
ResnetBlock(initial_filters * 8, strides=1),
ResnetBlock(initial_filters * 16, strides=2),
ResnetBlock(initial_filters * 16, strides=1),
])
self.final_bn = layers.BatchNormalization()
self.avg_pool = layers.GlobalMaxPool2D()
self.fc = layers.Dense(num_classes)
def call(self, inputs, training=None):
# print('x:',inputs.shape)
out = self.stem(inputs, training = training)
out = tf.nn.relu(out)
# print('stem:',out.shape)
out = self.blocks(out, training=training)
# print('res:',out.shape)
out = self.final_bn(out, training=training)
# out = tf.nn.relu(out)
out = self.avg_pool(out)
# print('avg_pool:',out.shape)
out = self.fc(out)
# print('out:',out.shape)
return out
def main():
num_classes = 5
resnet18 = ResNet(5)
resnet18.build(input_shape=(None, 224, 224, 3))
resnet18.summary()
if __name__ == '__main__':
main()
"""
"""
# 2.resnet18訓練, 圖片數量較小,訓練結果不是特別好
# resnet = ResNet(5) # 0.7607
# resnet.build(input_shape=(None, 224, 224, 3))
# resnet.summary()
"""
## 2.3 VGG19遷移學習
遷移學習利用了資料集之間的相似性,對於資料集數量較少的時候,訓練效果會遠優於其他。
在訓練過程中,使用include_top=False, 去掉最後分類的基層Dense, 重新構建並訓練就可以了。準確率0.9316
"""
# 3. VGG19遷移學習,遷移學習利用資料集之間的相似性, 結果遠好於其他2種
# 為了方便,這裡仍然使用resnet命名
net = tf.keras.applications.VGG19(weights='imagenet', include_top=False, pooling='max' )
net.trainable = False
resnet = keras.Sequential([
net,
layers.Dense(5)
])
resnet.build(input_shape=(None, 224, 224, 3)) # 0.9316
resnet.summary()
early_stopping = EarlyStopping(
monitor='val_loss',
patience=3,
min_delta=0.001
)
resnet.compile(optimizer=optimizers.Adam(lr=1e-3),
loss=losses.CategoricalCrossentropy(from_logits=True),
metrics=['accuracy'])
resnet.fit(db_train, validation_data=db_val, validation_freq=1, epochs=100,
callbacks=[early_stopping])
resnet.evaluate(db_test)
"""
附錄:
train_scratch.py 程式碼
"""
import os
os.environ['TF_CPP_MIN_LOG_LEVEL'] = '2'
import tensorflow as tf
import numpy as np
from tensorflow import keras
from tensorflow.keras import layers, optimizers, losses
from tensorflow.keras.callbacks import EarlyStopping
tf.random.set_seed(22)
np.random.seed(22)
assert tf.__version__.startswith('2.')
# 設定GPU視訊記憶體按需分配
# gpus = tf.config.experimental.list_physical_devices('GPU')
# if gpus:
# try:
# # Currently, memory growth needs to be the same across GPUs
# for gpu in gpus:
# tf.config.experimental.set_memory_growth(gpu, True)
# logical_gpus = tf.config.experimental.list_logical_devices('GPU')
# print(len(gpus), "Physical GPUs,", len(logical_gpus), "Logical GPUs")
# except RuntimeError as e:
# # Memory growth must be set before GPUs have been initialized
# print(e)
from pokemon import load_pokemon, normalization
from resnet import ResNet
def preprocess(x, y):
# x: 圖片的路徑,y:圖片的數字編碼
x = tf.io.read_file(x)
x = tf.image.decode_jpeg(x, channels=3) # RGBA
# 圖片縮放
# x = tf.image.resize(x, [244, 244])
# 圖片旋轉
# x = tf.image.rot90(x,2)
# 隨機水平翻轉
x = tf.image.random_flip_left_right(x)
# 隨機豎直翻轉
# x = tf.image.random_flip_up_down(x)
# 圖片先縮放到稍大尺寸
x = tf.image.resize(x, [244, 244])
# 再隨機裁剪到合適尺寸
x = tf.image.random_crop(x, [224, 224, 3])
# x: [0,255]=> -1~1
x = tf.cast(x, dtype=tf.float32) / 255.
x = normalization(x)
y = tf.convert_to_tensor(y)
y = tf.one_hot(y, depth=5)
return x, y
batchsz = 32
# create train db
images1, labels1, table = load_pokemon('pokemon', 'train')
db_train = tf.data.Dataset.from_tensor_slices((images1, labels1))
db_train = db_train.shuffle(1000).map(preprocess).batch(batchsz)
# create validation db
images2, labels2, table = load_pokemon('pokemon', 'valid')
db_val = tf.data.Dataset.from_tensor_slices((images2, labels2))
db_val = db_val.map(preprocess).batch(batchsz)
# create test db
images3, labels3, table = load_pokemon('pokemon', mode='test')
db_test = tf.data.Dataset.from_tensor_slices((images3, labels3))
db_test = db_test.map(preprocess).batch(batchsz)
# 1. 自定義小型網路
# resnet = keras.Sequential([
# layers.Conv2D(16, 5, 3),
# layers.MaxPool2D(3, 3),
# layers.ReLU(),
# layers.Conv2D(64, 5, 3),
# layers.MaxPool2D(2, 2),
# layers.ReLU(),
# layers.Flatten(),
# layers.Dense(64),
# layers.ReLU(),
# layers.Dense(5)
# ]) # 0.8547
# 2.resnet18訓練, 圖片數量較小,訓練結果不是特別好
# resnet = ResNet(5) # 0.7607
# resnet.build(input_shape=(None, 224, 224, 3))
# resnet.summary()
# 3. VGG19遷移學習,遷移學習利用資料集之間的相似性, 結果遠好於其他2種
net = tf.keras.applications.VGG19(weights='imagenet', include_top=False, pooling='max' )
net.trainable = False
resnet = keras.Sequential([
net,
layers.Dense(5)
])
resnet.build(input_shape=(None, 224, 224, 3)) # 0.9316
resnet.summary()
early_stopping = EarlyStopping(
monitor='val_loss',
patience=3,
min_delta=0.001
)
resnet.compile(optimizer=optimizers.Adam(lr=1e-3),
loss=losses.CategoricalCrossentropy(from_logits=True),
metrics=['accuracy'])
resnet.fit(db_train, validation_data=db_val, validation_freq=1, epochs=100,
callbacks=[early_stopping])
resnet.evaluate(db_test)