CNN 基礎之卷積及其矩陣加速
卷積在 CNN 中是非常基礎的一個操作, 但是, 一旦寫出來, 要畫不少的圖, 所以, 一直拖了下來, 剛好最近看到一個比較好的圖, 能夠說明卷積轉化為矩陣相乘就行操作的方法.
卷積操作的定義
卷積就是卷積核和另外一個矩陣 (圖片) 對應位置相乘然後結果相加.
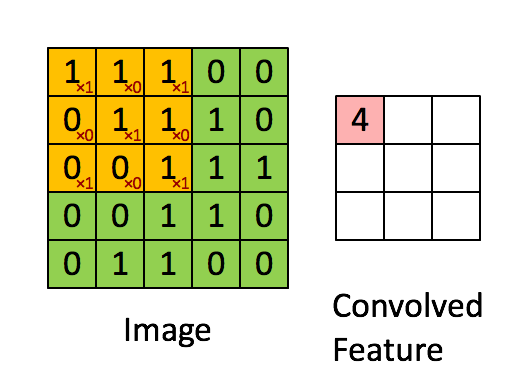
stride=1
的情形, 既每次移動一個畫素的位置, 根據需求, 也可以使用其他的stride
卷積計算的加速
對於大的卷積核, 加速方法一般是使用傅立葉變換 (或者其加強版: 快速傅立葉變換), 但是, 對於比較小的卷積核, 其轉換到頻域的計算量已經大於直接在空域進行卷積的計算量, 所以, 我們會發現在主流的深度學習框架中, 一般是直接在空域中進行卷積計算, 其加速計算的方法就是把卷積操作轉換成矩陣相乘 (因為有很多優化了的線性代數計算庫和 CUDA), 下面這張圖充分說明了具體過程 (2 維的情形).
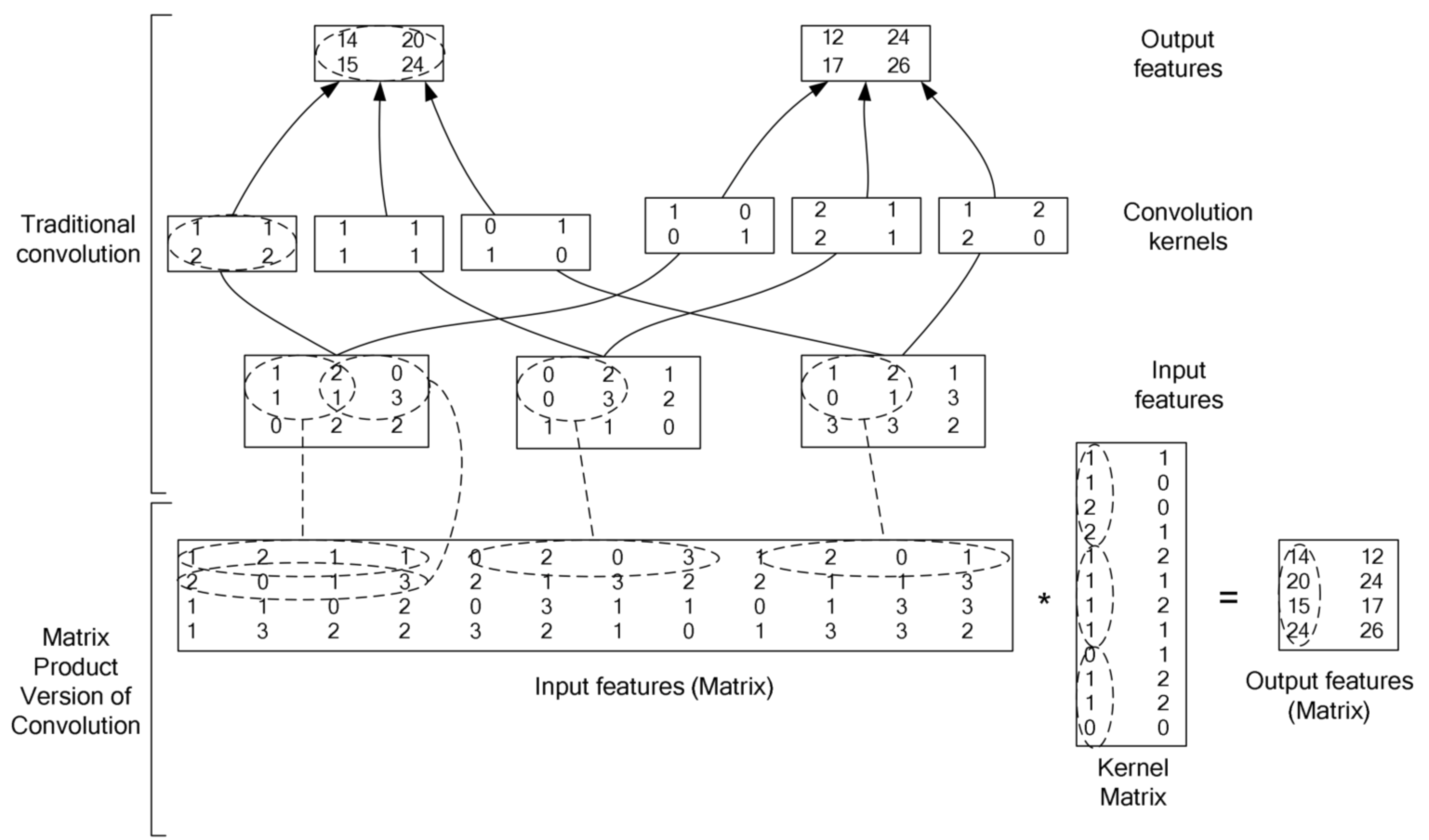
input features
im2col in Python
import numpy as np def get_im2col_indices(x_shape, field_height, field_width, padding=1, stride=1): # First figure out what the size of the output should be N, C, H, W = x_shape assert (H + 2 * padding - field_height) % stride == 0 assert (W + 2 * padding - field_height) % stride == 0 out_height = (H + 2 * padding - field_height) / stride + 1 out_width = (W + 2 * padding - field_width) / stride + 1 i0 = np.repeat(np.arange(field_height), field_width) i0 = np.tile(i0, C) i1 = stride * np.repeat(np.arange(out_height), out_width) j0 = np.tile(np.arange(field_width), field_height * C) j1 = stride * np.tile(np.arange(out_width), out_height) i = i0.reshape(-1, 1) + i1.reshape(1, -1) j = j0.reshape(-1, 1) + j1.reshape(1, -1) k = np.repeat(np.arange(C), field_height * field_width).reshape(-1, 1) return (k, i, j) def im2col_indices(x, field_height, field_width, padding=1, stride=1): """ An implementation of im2col based on some fancy indexing """ # Zero-pad the input p = padding x_padded = np.pad(x, ((0, 0), (0, 0), (p, p), (p, p)), mode='constant') k, i, j = get_im2col_indices(x.shape, field_height, field_width, padding, stride) cols = x_padded[:, k, i, j] C = x.shape[1] cols = cols.transpose(1, 2, 0).reshape(field_height * field_width * C, -1) return cols
參考連結