最小二乘法多項式曲線擬合原理與實現 zz
阿新 • • 發佈:2017-10-05
博客 del p s 並且 多項式 聯網 python mar 程序
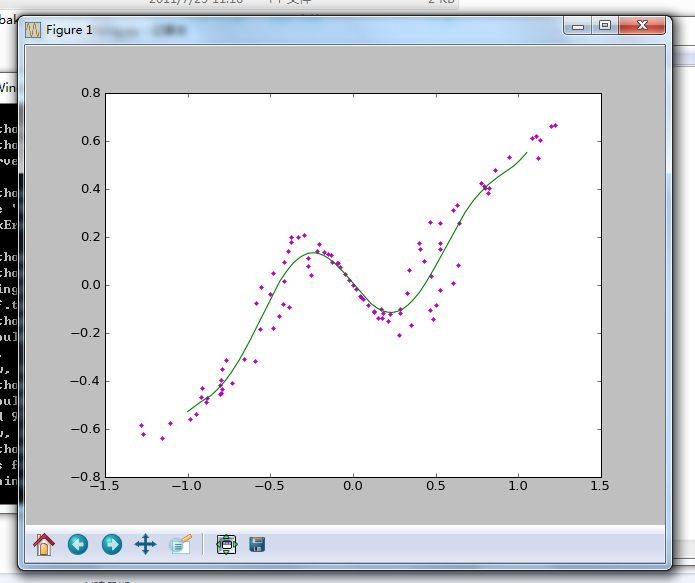
概念
最小二乘法多項式曲線擬合,根據給定的m個點,並不要求這條曲線精確地經過這些點,而是曲線y=f(x)的近似曲線y= φ(x)。
原理
[原理部分由個人根據互聯網上的資料進行總結,希望對大家能有用]
給定數據點pi(xi,yi),其中i=1,2,…,m。求近似曲線y= φ(x)。並且使得近似曲線與y=f(x)的偏差最小。近似曲線在點pi處的偏差δi= φ(xi)-y,i=1,2,...,m。
常見的曲線擬合方法:
1.使偏差絕對值之和最小
2.使偏差絕對值最大的最小
3.使偏差平方和最小
按偏差平方和最小的原則選取擬合曲線,並且采取二項式方程為擬合曲線的方法,稱為最小二乘法。
推導過程:
1. 設擬合多項式為:
2. 各點到這條曲線的距離之和,即偏差平方和如下:
.......
4. 將等式左邊進行一下化簡,然後應該可以得到下面的等式:
.......
5. 把這些等式表示成矩陣的形式,就可以得到下面的矩陣:
6. 將這個範德蒙得矩陣化簡後可得到:
7. 也就是說 運行前提:
- Python運行環境與編輯環境;
- Matplotlib.pyplot圖形庫,可用於快速繪制2D圖表,與matlab中的plot命令類似,而且用法也基本相同。
代碼:
[python] view plain copy- # coding=utf-8
- ‘‘‘‘‘
- 作者:Jairus Chan
- 程序:多項式曲線擬合算法
- ‘‘‘
- import matplotlib.pyplot as plt
- import math
- import numpy
- import random
- fig = plt.figure()
- ax = fig.add_subplot(111)
- #階數為9階
- order=9
- #生成曲線上的各個點
- x = numpy.arange(-1,1,0.02)
- y = [((a*a-1)*(a*a-1)*(a*a-1)+0.5)*numpy.sin(a*2) for a in x]
- #ax.plot(x,y,color=‘r‘,linestyle=‘-‘,marker=‘‘)
- #,label="(a*a-1)*(a*a-1)*(a*a-1)+0.5"
- #生成的曲線上的各個點偏移一下,並放入到xa,ya中去
- i=0
- xa=[]
- ya=[]
- for xx in x:
- yy=y[i]
- d=float(random.randint(60,140))/100
- #ax.plot([xx*d],[yy*d],color=‘m‘,linestyle=‘‘,marker=‘.‘)
- i+=1
- xa.append(xx*d)
- ya.append(yy*d)
- ‘‘‘‘‘for i in range(0,5):
- xx=float(random.randint(-100,100))/100
- yy=float(random.randint(-60,60))/100
- xa.append(xx)
- ya.append(yy)‘‘‘
- ax.plot(xa,ya,color=‘m‘,linestyle=‘‘,marker=‘.‘)
- #進行曲線擬合
- matA=[]
- for i in range(0,order+1):
- matA1=[]
- for j in range(0,order+1):
- tx=0.0
- for k in range(0,len(xa)):
- dx=1.0
- for l in range(0,j+i):
- dx=dx*xa[k]
- tx+=dx
- matA1.append(tx)
- matA.append(matA1)
- #print(len(xa))
- #print(matA[0][0])
- matA=numpy.array(matA)
- matB=[]
- for i in range(0,order+1):
- ty=0.0
- for k in range(0,len(xa)):
- dy=1.0
- for l in range(0,i):
- dy=dy*xa[k]
- ty+=ya[k]*dy
- matB.append(ty)
- matB=numpy.array(matB)
- matAA=numpy.linalg.solve(matA,matB)
- #畫出擬合後的曲線
- #print(matAA)
- xxa= numpy.arange(-1,1.06,0.01)
- yya=[]
- for i in range(0,len(xxa)):
- yy=0.0
- for j in range(0,order+1):
- dy=1.0
- for k in range(0,j):
- dy*=xxa[i]
- dy*=matAA[j]
- yy+=dy
- yya.append(yy)
- ax.plot(xxa,yya,color=‘g‘,linestyle=‘-‘,marker=‘‘)
- ax.legend()
- plt.show()
運行效果:
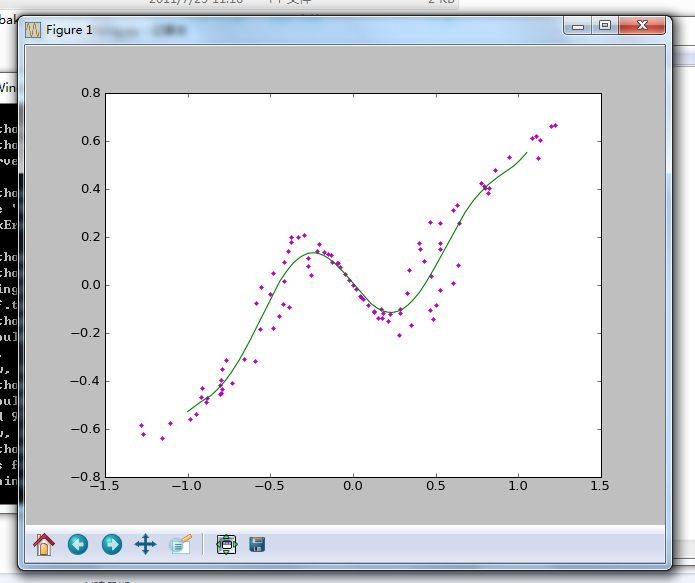
本博客中所有的博文都為筆者(Jairus Chan)原創。
如需轉載,請標明出處:http://blog.csdn.net/JairusChan。
如果您對本文有任何的意見與建議,請聯系筆者(JairusChan)。最小二乘法多項式曲線擬合原理與實現 zz